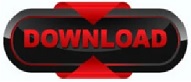
- #Rstudio 1.2 serial
- #Rstudio 1.2 series
The function takes on the arguments from, to and by which should be self-explanatory. In the first line we use a function called seq() to create the exact same vector as we did in the previous section, calling it z. 16.4 Volatility Clustering and Autoregressive Conditional Heteroskedasticity.16.2 Orders of Integration and the DF-GLS Unit Root Test.
#Rstudio 1.2 series
16 Additional Topics in Time Series Regression.15.6 Orange Juice Prices and Cold Weather.15.5 Estimation of Dynamic Causal Effects with Strictly Exogeneous Regressors.15.3 Dynamic Multipliers and Cumulative Dynamic Multipliers.15 Estimation of Dynamic Causal Effects.14.9 Can You Beat the Market? (Part II).14.6 Lag Length Selection Using Information Criteria.Forecast Uncertainty and Forecast Intervals.14.5 Additional Predictors and The ADL Model.Notation, Lags, Differences, Logarithms and Growth Rates.
#Rstudio 1.2 serial
14.2 Time Series Data and Serial Correlation.14.1 Using Regression Models for Forecasting.14 Introduction to Time Series Regression and Forecasting.The Differences-in-Differences Estimator.13.3 Experimental Estimates of the Effect of Class Size Reductions.13.2 Threats to Validity of Experiments.13.1 Potential Outcomes, Causal Effects and Idealized Experiments.12.5 Where Do Valid Instruments Come From?.12.4 Application to the Demand for Cigarettes.12.1 The IV Estimator with a Single Regressor and a Single Instrument.11.4 Application to the Boston HMDA Data.11.3 Estimation and Inference in the Logit and Probit Models.11.1 Binary Dependent Variables and the Linear Probability Model.11 Regression with a Binary Dependent Variable.10.6 Drunk Driving Laws and Traffic Deaths.10.5 The Fixed Effects Regression Assumptions and Standard Errors for Fixed Effects Regression.10.4 Regression with Time Fixed Effects.10.2 Panel Data with Two Time Periods: “Before and After” Comparisons.9.4 Example: Test Scores and Class Size.9.3 Internal and External Validity when the Regression is Used for Forecasting.9.2 Threats to Internal Validity of Multiple Regression Analysis.9 Assessing Studies Based on Multiple Regression.8.4 Nonlinear Effects on Test Scores of the Student-Teacher Ratio.
8.3 Interactions Between Independent Variables. 8.2 Nonlinear Functions of a Single Independent Variable. 8.1 A General Strategy for Modelling Nonlinear Regression Functions. 7.6 Analysis of the Test Score Data Set. Model Specification in Theory and in Practice. 7.5 Model Specification for Multiple Regression. 7.4 Confidence Sets for Multiple Coefficients. 7.3 Joint Hypothesis Testing Using the F-Statistic. 7.2 An Application to Test Scores and the Student-Teacher Ratio. 7.1 Hypothesis Tests and Confidence Intervals for a Single Coefficient. 7 Hypothesis Tests and Confidence Intervals in Multiple Regression. 6.5 The Distribution of the OLS Estimators in Multiple Regression. Simulation Study: Imperfect Multicollinearity. 6.4 OLS Assumptions in Multiple Regression. 6.3 Measures of Fit in Multiple Regression. 6 Regression Models with Multiple Regressors. 5.6 Using the t-Statistic in Regression When the Sample Size Is Small. Computation of Heteroskedasticity-Robust Standard Errors. Should We Care About Heteroskedasticity?. A Real-World Example for Heteroskedasticity. 5.4 Heteroskedasticity and Homoskedasticity. 5.3 Regression when X is a Binary Variable. 5.2 Confidence Intervals for Regression Coefficients. 5.1 Testing Two-Sided Hypotheses Concerning the Slope Coefficient. 5 Hypothesis Tests and Confidence Intervals in the Simple Linear Regression Model. 4.5 The Sampling Distribution of the OLS Estimator. Assumption 3: Large Outliers are Unlikely. Assumption 2: Independently and Identically Distributed Data. Assumption 1: The Error Term has Conditional Mean of Zero. 4.2 Estimating the Coefficients of the Linear Regression Model. 3.7 Scatterplots, Sample Covariance and Sample Correlation. 3.6 An Application to the Gender Gap of Earnings. 3.5 Comparing Means from Different Populations. 3.4 Confidence Intervals for the Population Mean. Hypothesis Testing with a Prespecified Significance Level. Calculating the p-value When the Standard Deviation is Unknown. Sample Variance, Sample Standard Deviation and Standard Error. Calculating the p-Value when the Standard Deviation is Known. 3.3 Hypothesis Tests Concerning the Population Mean. Large Sample Approximations to Sampling Distributions. 2.2 Random Sampling and the Distribution of Sample Averages. Probability Distributions of Continuous Random Variables. Probability Distributions of Discrete Random Variables.
2.1 Random Variables and Probability Distributions. 1.2 A Very Short Introduction to R and RStudio.